Newly Discovered Networks among Different Diseases Reveal Hidden Connections
Enormous databases of medical records have begun to reveal connections among diseases that could provide insights into the biological missteps that make us sick.
Stefan Thurner is a physicist, not a biologist. But not long ago, the Austrian national health insurance clearinghouse asked Thurner and his colleagues at the Medical University of Vienna to examine some data for them. The data, it turned out, were the anonymized medical claims records — every diagnosis made, every treatment given — of most of the nation, which numbers some 8 million people. The question was whether the same standard of care could continue if, as had recently happened in Greece, a third of the funding evaporated. But Thurner thought there were other, deeper questions that the data could answer as well.
In a recent paper in the New Journal of Physics, Thurner and his colleagues Peter Klimek and Anna Chmiel started by looking at the prevalence of 1,055 diseases in the overall population. They ran statistical analyses to uncover the risk of having two diseases together, identifying pairs of diseases for which the percentage of people who had both was higher than would be expected if the diseases were uncorrelated — in other words, a patient who had one disease was more likely than the average person to have the other. They applied statistical corrections to reduce the risk of drawing false connections between very rare and very common diseases, as any errors in diagnosis will get magnified in such an analysis. Finally, the team displayed their results as a network in which the diseases are nodes that connect to one another when they tend to occur together.
The style of analysis has uncovered some unexpected links. In another paper, published on the scientific preprint site arxiv.org, Thurner’s team confirmed a controversial connection between diabetes and Parkinson’s disease, as well as unique patterns in the timing of when diabetics develop high blood pressure. The paper in the New Journal of Physics generated additional connections that they hope to investigate further.
Eventually, Thurner and a growing number of other researchers hope to use these disease networks to generate hypotheses about how diseases operate at the molecular level. “Is this disease caused by a gene?” Thurner said. “Is it caused by a defect in the metabolic network? Is it due to environmental things that affect certain genes? Things like this. This is the aim.”
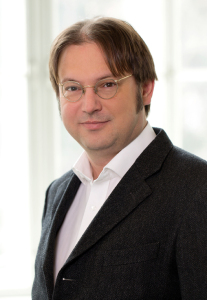
Medical University of Vienna/Matern
Stefan Thurner analyzed the anonymized medical records of all of Austria.
The work is being driven by the realization that diseases, as defined in medicine, sound like tidy, distinct entities, but are messier in reality. Diseases tend to be defined by their symptoms. But the molecular roots of a disease may have biological effects that go far beyond our current understanding. Certain diseases tend to follow others or have high rates of comorbidity, and though it isn’t clear why, it may be because they arise from related biological flaws.
“The idea is, connections at the cellular level get amplified at the population level, and they emerge as comorbidity,” said Albert-László Barabási, a physicist at Northeastern University who has published several landmark papers in this area, including a 2009 article in PlOS Computational Biology that helped inspire Thurner, as well as a 2011 review of the field in Nature Reviews Genetics. Using a disease network, a researcher might suggest that biologists look for new disease genes shared between diseases one and two, for instance, where there seems to be a strong connection.
Biologists typically look for genetic connections by using genome-wide association studies, which statistically associate genetic markers with disease. But at Harvard Medical School, another research team is attempting to find the same connections by mapping networks of a very different kind: the molecular networks at work in a cell.
Networks of Life
The inside of a cell seethes with activity, as tiny molecules, enormous proteins and strands of DNA wash around each other going about their business. Each actor’s business is some set of other actors — a protein, for instance, might snip pieces off of other proteins, ferry molecules around, or jump-start the manufacturing of DNA. It takes its cues from other actors, which can make it work faster or more slowly or send it off to distant regions where it’s needed.
The functioning of the cell can take on a very different character if even a single member of this molecular social network starts to behave oddly. Before long, the effects ripple outward from the initial flaw, causing problems — disease — on the level of the organism. A disease is in some sense just an expression of the underlying dynamics of this social structure. Thurner hopes his disease networks can eventually help uncover some of these flaws.
And it’s here at the sub-microscopic end of things that Joseph Loscalzo, a professor at Harvard Medical School and a long-time collaborator of Barabási’s, is mapping his own network. He and his team start by gleaning data from numerous databases on which proteins interact with each other and how. Then, using a computer model, they sketch out the social network within an average cell, connecting individual genes and proteins to one another if they happen to interact. Loscalzo’s team has built a diagram with 13,460 protein nodes and 141,296 links. (These interactions probably account for only about 20 to 25 percent of the total, Loscalzo says, but it’s a start.) Then they isolate just the nodes that have been statistically linked to a given disease. They call this set of nodes the disease module.
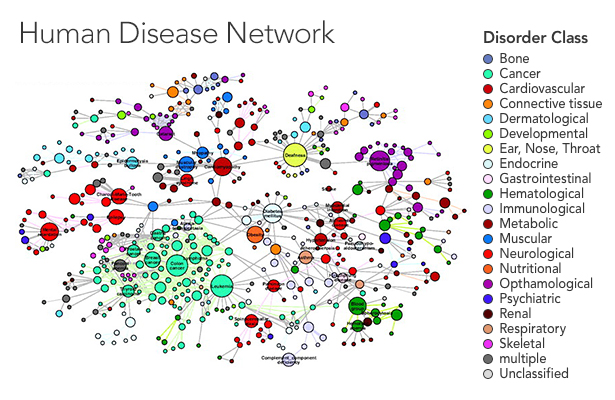
Olena Shmahalo/Quanta Magazine; source: Albert-László Barabási
A human disease network maps out connections between diseases — if patients who have one disease tend to also have another, the two disease nodes are connected.
One disease module they’ve studied is for pulmonary hypertension — high blood pressure in the lungs, which can cause heart failure. They looked at all the molecular pathways that genome-wide association studies suggested were involved. They then studied which pathways grow more active in animal models and in pulmonary hypertension patients under stress. Their disease module revealed that two proteins previously linked to some forms of the disease were part of the same molecular pathway and that they work together to cause errors in cell proliferation, which may be linked to the symptoms of the disease. The researchers published their findings in the journal Pulmonary Circulation.
Another module looks at Type 2 diabetes. Researchers have linked diabetes to about 200 spots on the genome through genome-wide association studies. “The first 18 or so of those are highly significant, but the last 182 or so are just at the margin,” Loscalzo said. But in the disease module, it was clear that some of those 182 genes were highly connected hubs in the social network, a state of affairs that a genome-wide association study alone is not equipped to reveal. “We’ve explored three of those [genes] now, and they highlight pathways that had been peripherally believed to be associated with diabetes but never demonstrated in any careful way,” he said.
Combining Loscalzo’s molecular networks with Thurner and Barabási’s disease networks would help to create a bridge between correlation and mechanism. If comorbid diseases share overlapping molecular networks, researchers could use the networks to understand the biochemical mechanisms behind them. These two kinds of networks, very different in how they are built, are united only by the idea that data can reveal connections that otherwise would pass unnoticed. But together these networks have the potential to open new doors in the study of disease.
“Once you draw a network, you are drawing hypotheses on a piece of paper,” Thurner said. “You are saying, ‘Wow, look, I didn’t know these two things were related. Why could they be? Or is it just that our statistical threshold did not kick it out?’” In network analysis, you first validate your analysis by checking that it recreates connections that people have already identified in whatever system you are studying. After that, Thurner said, “the ones that did not exist before, those are new hypotheses. Then the work really starts.”
It is worth remembering that both techniques are still relatively new. Loscalzo can reel off ways that his results could be flawed — the sprawling incompleteness of the data on protein-protein interactions is a major concern, but so are the methods used to gather the data, which are the best currently possible but far from perfect. And Thurner and his students are still gathering collaborators in biology who can test their hypotheses. After they published their first results from the database a couple of years ago, Thurner said wryly, “we thought we would have a hundred people sitting in our office,” looking to collaborate. So far, the response has been more of a trickle.
“It’s not uncontroversial,” said Andrey Rzhetsky, a professor of genetics at the University of Chicago with a background in mathematical biology who has published on comorbidity networks. “Some people feel very strongly about big data sets — almost to the point of fanatic refusal to accept results from large-scale analysis.” The argument, he explains, is that there are unknown biases in large data sets. In the case of databases like Thurner’s, these biases stem from the different ways doctors enter information into medical records, the way ethnicity is accounted for, and so on. Rzhetsky acknowledges the danger of biases but believes they do not eliminate the usefulness of the data, provided researchers are careful with their interpretations. “I do think it’s the direction for the future, but it’s far from a solved problem,” he said. He was intrigued by the article in the New Journal of Physics. “The model is extremely simple, but the direction is great,” he wrote in an email.
Loscalzo is aware of his colleagues’ scrutiny. “When I give talks about network medicine,” he said, “I’ve gotten three kinds of responses. At one end of the spectrum are generally young people … who say this is a great idea, I hadn’t thought about this before. … At the other end of the spectrum I have people my age or older who say: ‘What are you talking about? I’m a member of the National Academy and that’s all based on reductionist biology, I’m not going to change my strategy.’ Then in the middle you’ve got this broad swath of people who have a healthy skepticism and who want there to be some sort of proof that these notions can give us new insights. And that’s what we’ve been working on.”
No comments:
Post a Comment